原文信息:
MEBA: AI-powered precise building monthly energy benchmarking approach
原文链接:
https://www.sciencedirect.com/science/article/pii/S0306261924000990
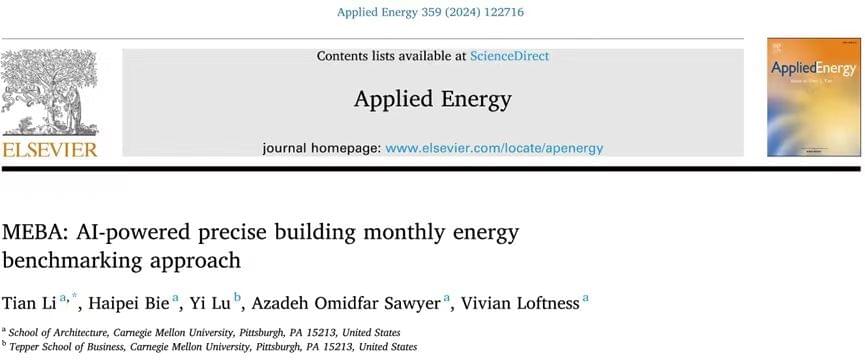
Highlights
• 通过基于能源使用模式的智能聚类模型对建筑进行分类。
• 通过部分月度或全年度能源数据场景预测月度能源使用量。
• 建筑类型与能源使用模式之间没有显著相关性。
• 能源使用强度(EUI)、电力使用和建筑年份是能源预测的关键属性。
• 在分类、解释性和预测方面超越了现有最先进的模型。
Research Gap
MEBA旨在以前所未有的精度和灵活性应对建筑能源管理的复杂性。借助MEBA,利益相关者(业主、管理者和政策制定者)可以通过导入年度总量或部分月度能耗来获取精确的月度能源数据。这使他们能够为未来的能源和运营成本节省做出更好的决策,以尽可能少的资本实现碳中和。
摘要
建筑月度能源基准比年度或小时基准更好地支持识别趋势、提高能源效率以及为建筑业主、管理者和政策制定者的成本管理。年度数据无法全面反映运营效用状况,而每小时数据又存在规模较小,数据挖掘成本高、不可比等问题。然而,月度能源基准的主要挑战是数据限制、算法“黑匣子”障碍和建筑分类不确定。这项研究提出了一种新颖的人工智能驱动的月度能源基准法(MEBA),以更好地评估建筑能源使用模式、评估电能及燃气终端荷载并跟踪能耗费用账单。MEBA 可以解决两种情况:(1)使用部分月度能源数据预测全年月度能源总量;(2) 根据年度总能源数据估算每月能耗。该研究收集了美国两个主要城市逐月的电力和天然气能源使用情况。对于第一种情况,整个数据集通过高斯混合模型(GMM)聚类为两个主要组。然后,通过自组织映射(SOM)模型通过能源使用模式将这两个组划分为五个子簇。对于第二种情况,则需要执行集成学习LGBM分类模型来定位子簇标签。所有五个子簇都具有很精准的预测性能,平均准确度>95%。这两种情况都需要进行最后阶段通过 LGBM 回归模型来预测每月的电力和天然气能耗。MEBA的预测性能实现 R2 范围为 0.50 至 0.73,RMSE 范围为 0.15 至 2.35,明显优于最先进的 XGBoost 模型。每个子集群都表现出不同的能源使用特点,其中能源使用强度、电力负荷和建造年份是影响月能耗的最重要属性。
更多关于"AI-powered"的研究详见:
https://www.sciencedirect.com/search?qs=AI-powered&pub=Applied%20Energy&cid=271429
Abstract
Monthly energy benchmarking supports identifying trends, improving energy efficiency, and conducting cost management for building owners, managers, and policymakers better than annual or hourly benchmarking. Annual data cannot fully reflect operation utility status, and hourly data poses the issue of high-cost data mining and incomparability due to its minor scale. However, the primary challenges of monthly energy benchmarking are data limitation, “black-box” barrier, and building classification uncertainty. This study proposes a novel AI-powered Monthly Energy Benchmarking Approach (MEBA) to better assess building energy use patterns, benchmark end-use loads, and track utility bills. MEBA addresses two scenarios: (1) predict complete year-round monthly energy using partial monthly energy data; (2) estimate monthly energy loads from annual total energy data. The study collects monthly electricity and natural gas energy use from two U.S. cities. For the first scenario, the entire dataset is clustered into two primary groups by Gaussian Mixture Model (GMM). Then, the two groups are divided by Self-Organizing Map (SOM) models into five subclusters via energy use patterns. For the second scenario, an additional step is needed to locate the subcluster labels with advanced Light Gradient Boosting Machine (LGBM) classifications. All five subclusters have high prediction performance with an average accuracy of >95%. Both scenarios require the last stage to predict monthly electricity and natural gas by LGBM regressions. MEBA's prediction performance achieves R2s ranging from 0.50 to 0.73, with RMSEs between 0.15 and 2.35, outperforming the state-of-the-art XGBoost model. Each subcluster exhibits distinct energy use patterns, with EUIs, electricity loads, and year built as the most significant attributes.
Keywords
Energy benchmarking
Monthly energy use
Building classification
AI-driven method
Unsupervised clustering
Supervised learning
Graphics
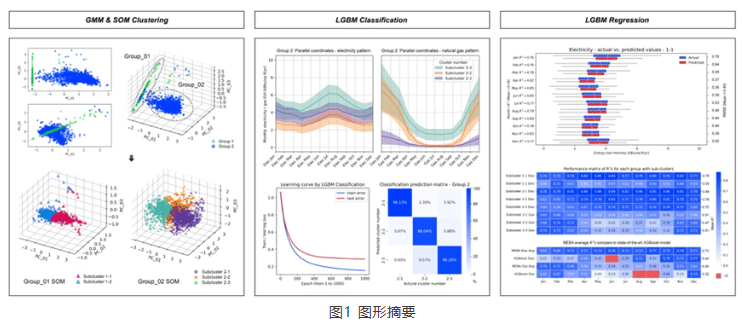
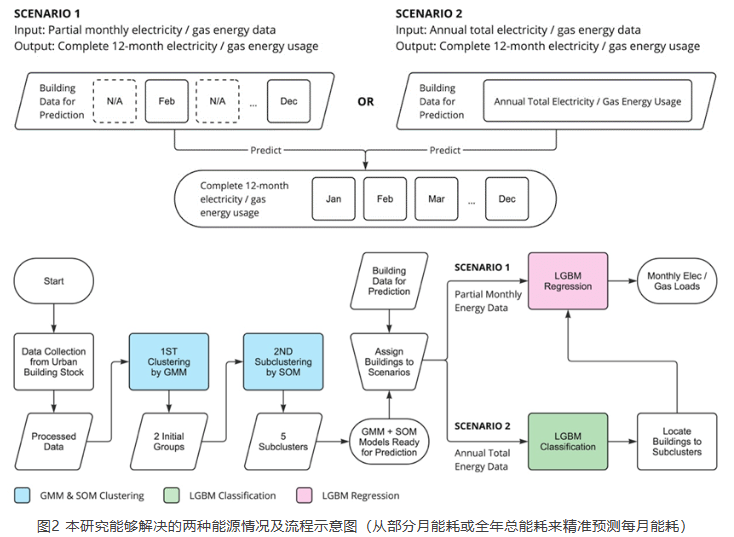
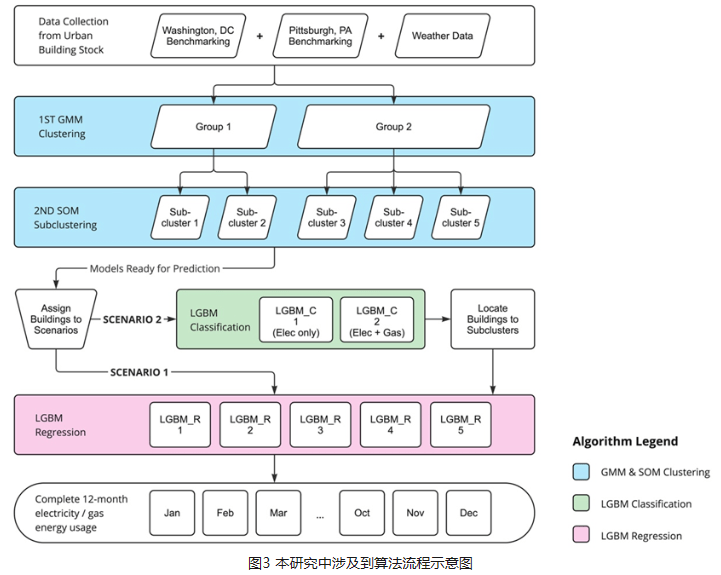
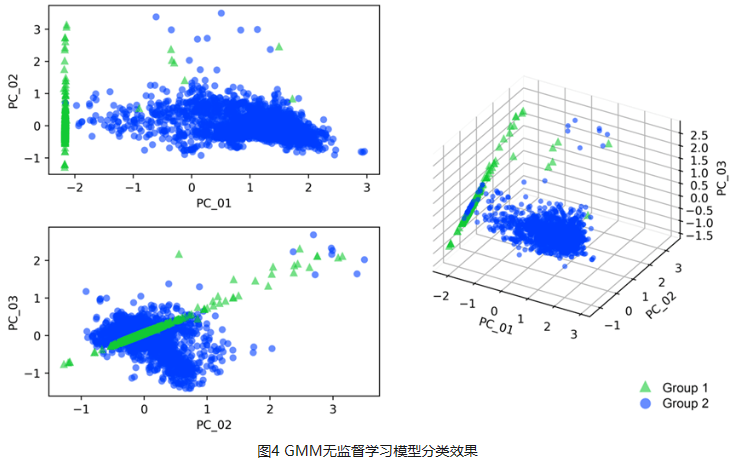
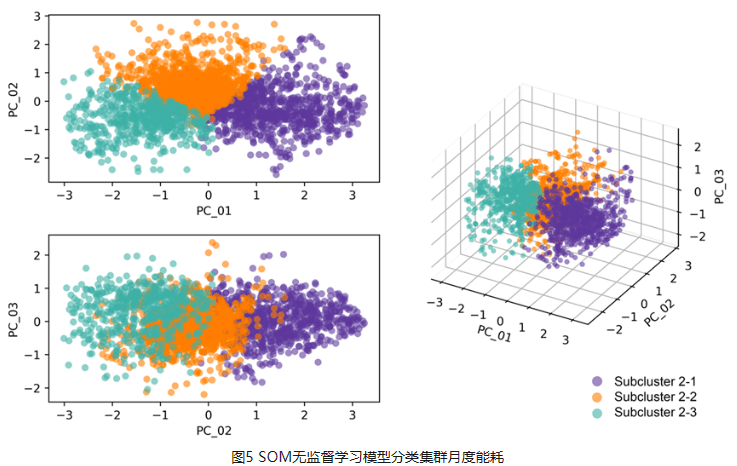
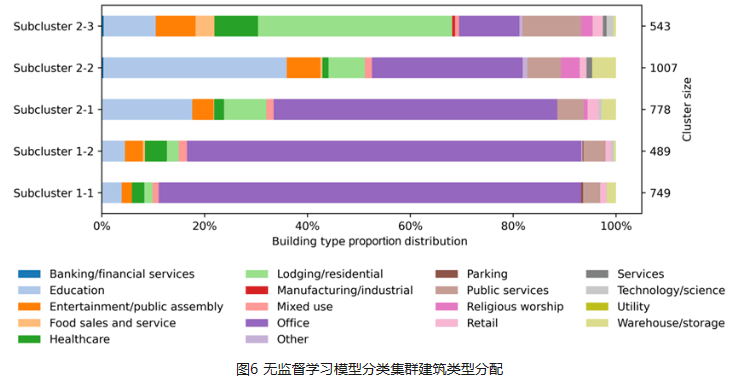
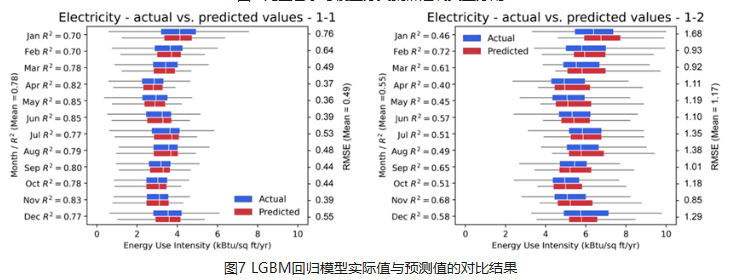
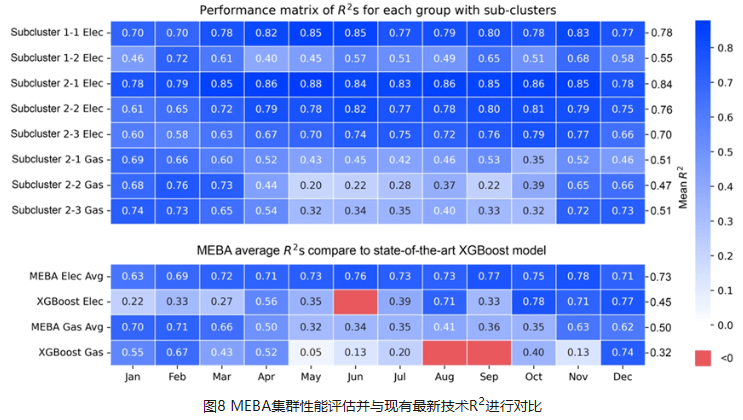
团队介绍
本研究由美国卡内基梅隆大学(Carnegie Mellon University)建筑学院与商学院的研究人员合作完成。其中包含:李恬(博士生,客座教授)、别海培(博士生,教学/科研助理)、陆祎(建筑师,MBA)、Azadeh O. Sawyer(建筑采光专家,助理教授,博士生导师)和Vivian Loftness(建筑性能与绿建专家,教授,博士生导师)。
通信作者/第一作者简介:
李恬,美国卡内基梅隆大学(Carnegie Mellon University)博士研究生,匹兹堡大学(University of Pittsburgh)客座教授,LEED认证专家,建筑设计师,从事综合建筑设计,人工智能驱动建筑能耗与建筑舒适度,能源转型与气候韧性等领域研究。获得美国圣路易斯华盛顿大学(Washington University in St. Louis)和天津大学建筑学院双硕士。在Applied Energy、Developments in the Built Environment等顶尖SCI期刊和ASHRAE国际建筑大会发表多篇一作论文,并多次受邀报告与讲座。